Data Sciences Institute Reproducibility Thematic Program
The growing use of large-scale complex data across disciplines has brought the challenge of reproducibility to the forefront. But how can we foster trust in data-informed research? The Data Sciences Institute (DSI) Reproducibility Thematic Program aims to address such questions by focusing on the development of widely adoptable methodology and processes to share data and code, as well as the development of infrastructure, methods, and models that support reproducible and reliable research. Ambitions for the program include educating the next generation of researchers on the importance of transparency, removing the intimidation factor from reproducibility, and supporting researchers in identifying their path to reproducibility.
“There is a lot of discussion about how the lack of reproducible results may make people question their confidence in science. We saw this play out during the pandemic. Robust and reproducible processes are paramount to maintaining confidence in the research enterprise and ensuring the generation of reliable results upon which science builds. We want to push to make the DSI a centre for reproducible science, and help researchers understand how to adopt best practices in reproducibility,” says Timothy Chan, DSI associate director of research and thematic programming.
Last fall, the DSI held an open call for researchers to co-lead this thematic program. Reproducibility co-leads Professors Rohan Alexander, Benjamin Haibe-Kains and Jason Hattrick-Simpers represent different research fields– from social sciences to life and physical sciences – but they are united in their passion for supporting and advocating for transparency and reproducibility in research. They are in the process of developing programs and community-building activities.
Stay tuned for Reproducibility activities and opportunities.
2022 Toronto Workshop on Reproducibility
The Reproducibility Thematic Program kicked off with a multi-day workshop that brought together over 460 academic, industry, and non-profit participants on the critical issue of reproducibility in applied statistics and related areas. The workshop was hosted by the DSI and CANSSI Ontario.
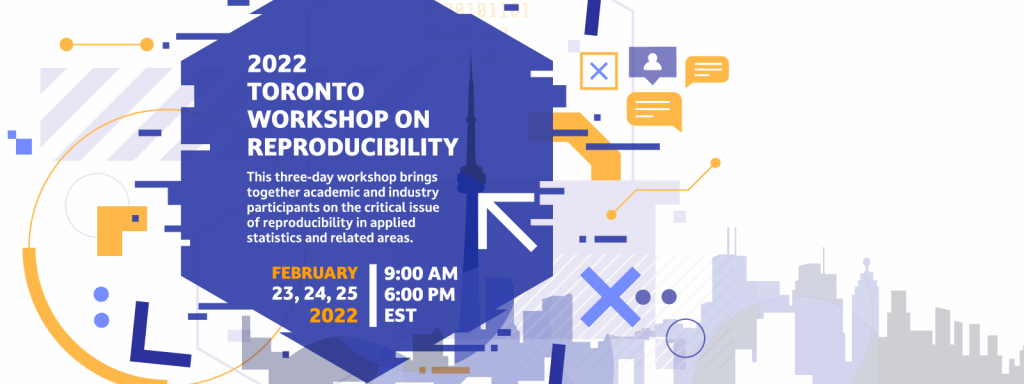
Topics at the workshop ranged from reproducibility in language modelling and machine learning, to biomedical research, to integrating reproducibility in undergraduate social science programs and reproducibility in crowd science.
The workshop featured over forty speakers from the University of Toronto, University Health Networks, Canadian and International research universities and focused on evaluating and teaching reproducibility, as well as reproducibility practices. “We were tremendously pleased with the caliber of the speakers,” says Rohan Alexander, the lead organizer. “The deep engagement speaks to the importance of reproducibility. To create understanding it is important that others can trust results.”
Reproducibility champion and world-renowned computer scientist, Professor Joëlle Pineau, spoke to improving reproducibility in machine learning research. “Reproducibility is a minimum necessary condition for a finding to be believable and informative,” says Pineau, an associate professor at the School of Computer Science at McGill University. She co-directs the Reasoning and Learning Lab at McGill and also leads the Facebook AI Research lab.
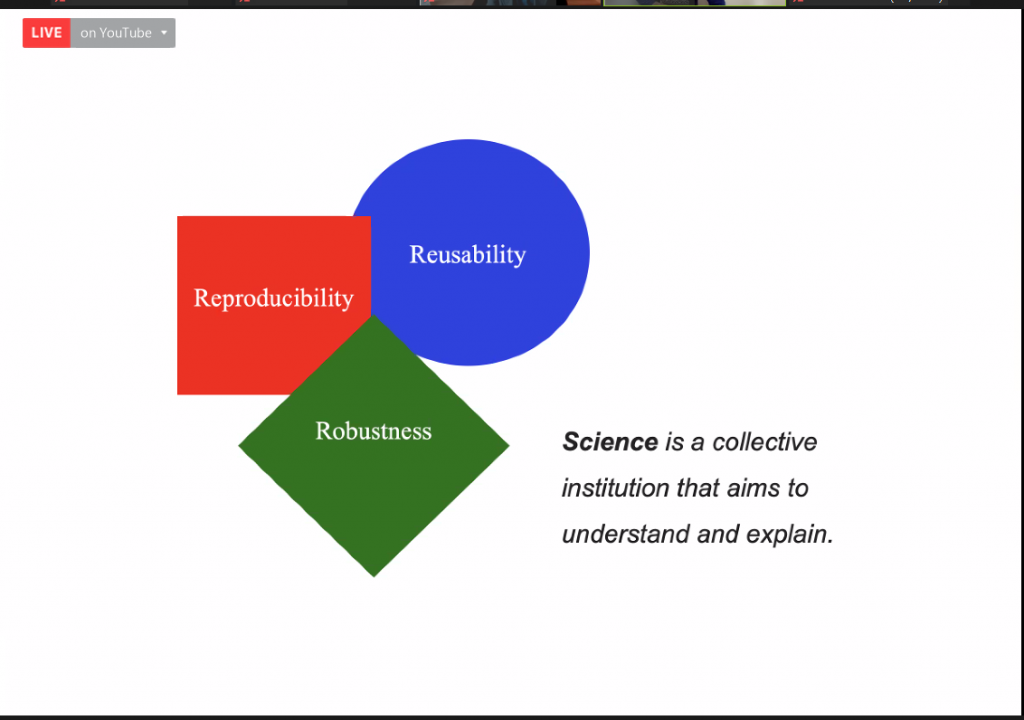
Professor Colm-Cille Patrick Caulfield from the University of Cambridge discussed why an honest discussion of uncertainty in models is critical for climate science. “We have such a complex climate system, only by ensuring transparency can we be 100% confident in our predictions,” Caulfield says. The DSI and the C2D3 Cambridge Centre for Data-Driven Disc are planning to host joint workshops around the DSI’s Thematic Programs of Inequity and Reproducibility.
Meet the Reproducibility Co-Leads
The DSI is excited to announce co-leads for its Thematic Program in Reproducibility. The co-leads are responsible for the thematic program events, activities, and community-building.
Rohan Alexander is an assistant professor at the Faculty of Information and Department of Statistical Sciences at the University of Toronto. He is the assistant director of CANSSI Ontario, a senior fellow at Massey College, and a faculty affiliate at the Schwartz Reisman Institute for Technology and Society. He is interested in using statistics to understand the world.
“I am particularly interested in how we turn something as complicated as society into a dataset that can be analyzed, and what we lose in exchange for the benefits that such statistical modeling brings. I applied to be a Reproducibility co-lead to contribute to the improvements that are happening in the social sciences and to share and learn from other disciplines.”
Benjamin Haibe-Kains is a senior scientist with the University Health Network and an Associate Professor of Medical Biophysics at the Temerty Faculty of Medicine. His research program focuses on developing multimodal models, using radiological images and large-scale genomic data, to predict the survival and therapy response of cancer patients.
“I have always been passionate about research transparency and reproducibility, key components of Open Science. When I saw that the newly created Data Sciences Institute had an open call for leading their Reproducibility Theme, I could not miss this unique opportunity to educate on how to make research more transparent and reproducible.”
Jason Hattrick-Simpers is a Professor at the Department of Materials Science and Engineering, at the University of Toronto and a Research Scientist at CanmetMATERIALS. His research focuses on the creation of tools to enable the discovery of new corrosion-resistant materials or new materials for converting waste heat into usable energy.
“Reproducibility is at the heart of the scientific method and is what allows us to live in a world filled with technological wonders.”