By Kate Baggott
“Causal inference is hard.”
That’s not a conclusion. It’s an observation Rahul G. Krishnan was brave enough to make at the Forging a Path: Causal Inference and Data Science for Improved Policy Workshop on November 10th to over 100 faculty, students and participants from organizations.
The difficulty of causal inference is not a matter of methodological rigour or reporting. The difficulty comes from the interdisciplinary nature of the process. The community doing causal inference is not one community, Krishnan reminded those present. Rather, causal inference is a process that engages different communities; biostatisticians, economists, epidemiologists, computer scientists, and data scientists, among others; engage in to make decisions and form policies.
“Among these communities, different language is used to describe the same phenomenon,” Krishnan said.”
The workshop was created to bring together practitioners of multiple disciplines who are employing a variety of methodologies. The Data Science Institute funds the Causal Inference Emerging Data Science Program and held the workshop in collaboration with the Forward Society (FOS) Lab. The program was initiated by University of Toronto‘s Linbo Wang (Department of Statistical Sciences, University of Toronto Scarborough), Gustavo J. Bobonis (Department of Economics, Faculty of Arts & Science), Ismael Mourifié (Department of Economics, Faculty of Arts & Science), and Raji Jayaraman (Department of Economics, Faculty of Arts & Science). The workshop was the first of three workshops and a seminar series over the new two-years of the emerging data science program.
The challenge put to participants was not to create a common language, but to create a shared understanding for how to manage the reams of data collected on human activity and explain it to help policymakers improve their decision-making in all areas from public health to education, and from social security to law and justice.
Throughout the presentations from practitioners, there was an emphasis on description, shared definitions, and clear communication when working with decision-makers.
Econometrician and empirical microeconomist Alberto Abadie (MIT Economics) talked about estimating the value of evidence-based decision-making (EBDM) itself in his keynote presentation.
“Despite the ubiquity of EBDM, we are unaware of empirical tools that organizations can use to assess the value of their EBDM practices,” he reminded attendees of the workshop. “Part of the challenge in evaluating the value of EBDM is that it requires a description of what organizations will do with and without various amounts of evidence that they can choose to generate at some cost.”
Professor Elizabeth Halloran (Fred Hutchinson Cancer Center) is a world leader in using mathematical and statistical methods to study infectious diseases and a pioneer in the design and analysis of vaccine studies.
“Important examples of global public health policies where causal inference with interference can make a difference include vaccines and vaccination programs,” she reminded participants.
Causal estimates demonstrating indirect effects of intervention programs, she said, can make policies in all fields more cost-effective.
The workshop concluded with a student-led roundtable discussion where Vahid Balazadeh, Sonia Markes, Stephen Tino, Dario Toman, and Atom Vayalinkal outlined next steps in the efforts to bring together causal inference and data sciences communities.
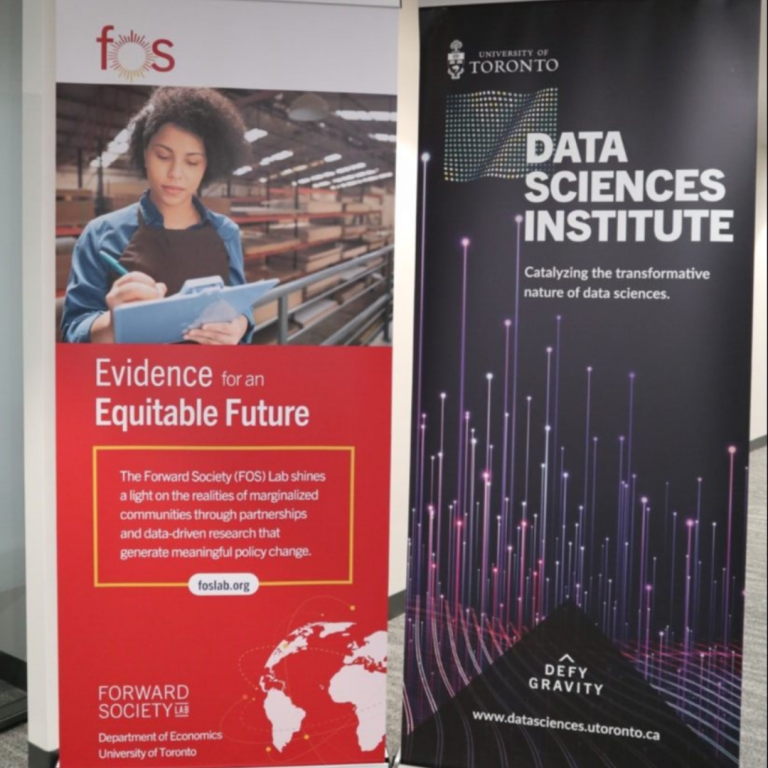
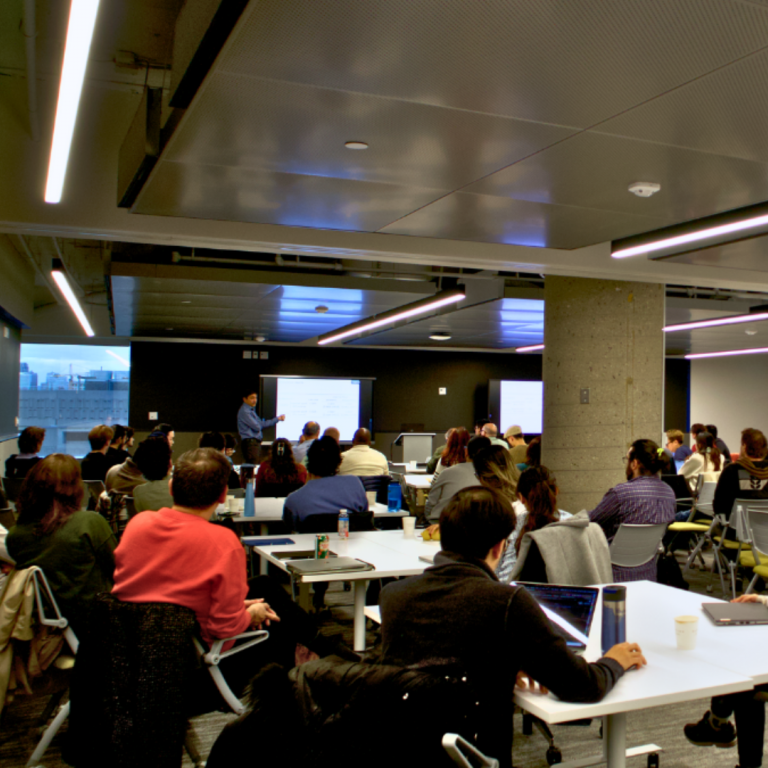
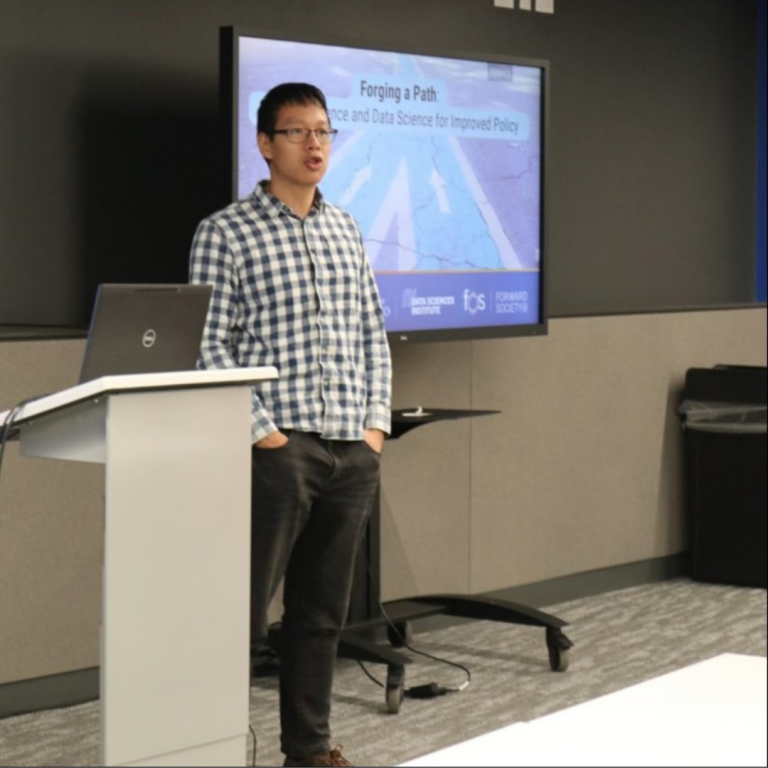
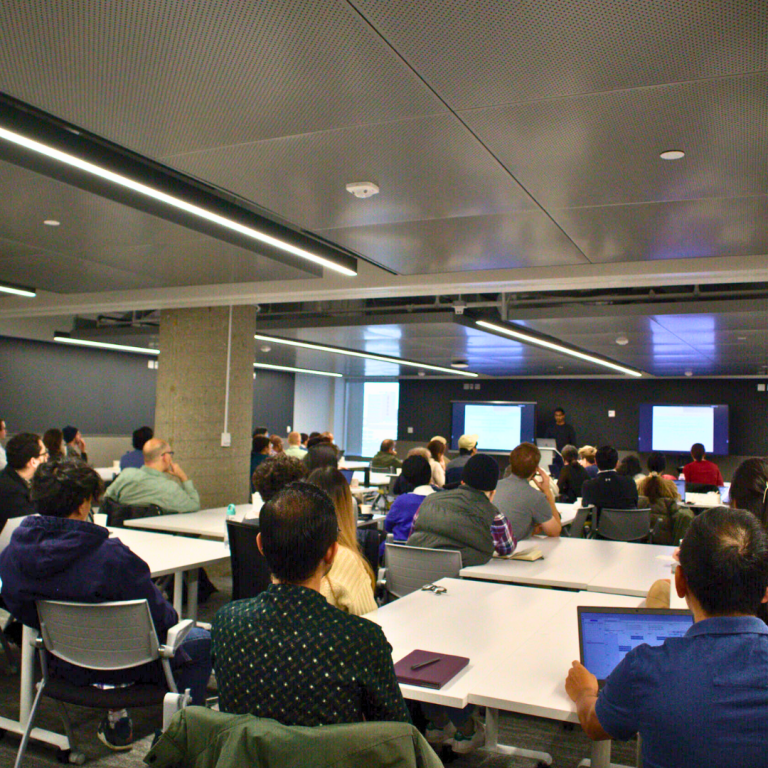

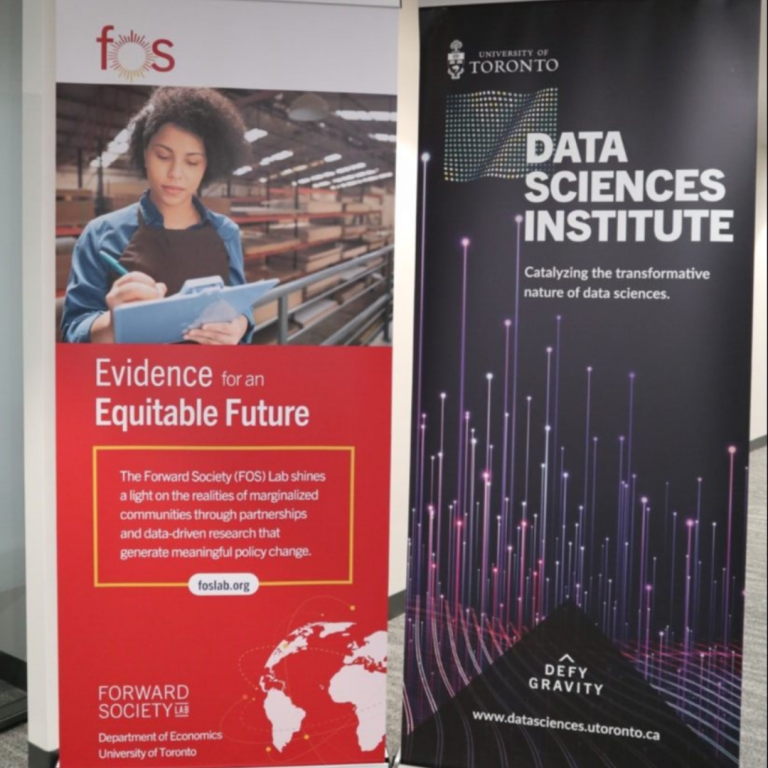
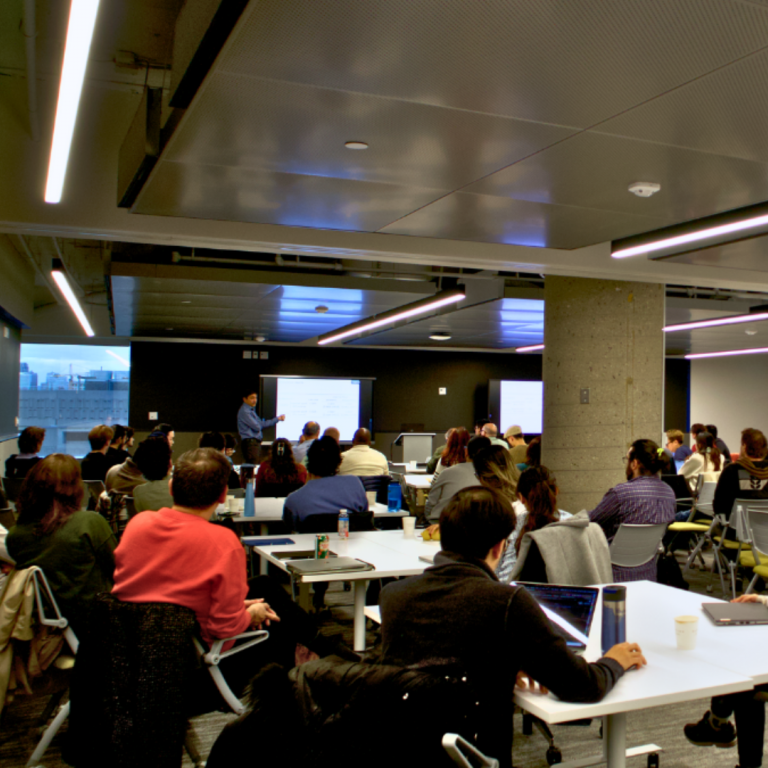