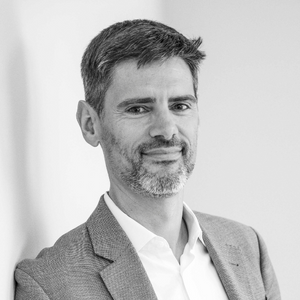
Without causal inference, AI is FI (fake intelligence)- Prof. Miguel Hernan
Join us for the Data Sciences Speaker Series with Prof. Miguel Hernan, Director of CAUSALab and Professor, Biostatistics and Epidemiology, Harvard T.H. Chan School of Public Health. This talk is co-sponsored by the Data Sciences Institute and Dalla Lana School of Public Health at the University of Toronto.
Registration Required – REGISTER HERE
- Date: February 28, 2023
- Time: 11:00 AM – 12:00 PM ET
- Format: In-person only
Talk Title: Without causal inference, AI is FI (fake intelligence)
Description: The word “artificial” may be interpreted as “made by humans” (as in artificial flavor) or as “fake” (as in artificial smile). In this talk, I will argue that the second meaning is more appropriate for AI in 2023. A combination of clever algorithms, large amounts of data, and increasingly powerful computers has led to impressive advances in so-called AI. However, in the health and social sciences, these advances are largely concerned with tasks that use data for prediction (e.g., pattern recognition, curve fitting) rather than for counterfactual prediction. The latter is a key feature of intelligent beings and the basis of causal inference. When dealing with the complex systems of the health and social sciences, counterfactual prediction based on the integration of data and causal models remains a future goal for AI agents.
About the speaker: Miguel Hernán uses health data and causal inference methods to learn what works. As Director of the CAUSALab at Harvard, he and his collaborators repurpose real world data into scientific evidence for the prevention and treatment of infectious diseases, cancer, cardiovascular disease, and mental illness. As the Kolokotrones Professor of Biostatistics and Epidemiology, he teaches at the Harvard T.H. Chan School of Public Health, where he has mentored dozens of trainees and students, and at the Harvard-MIT Division of Health Sciences and Technology. His free online course “Causal Diagrams” and book “Causal Inference: What If”, co-authored with James Robins, are widely used for the training of researchers. Miguel has received many awards for his work, including the Rousseeuw Prize for Statistics, the Rothman Epidemiology Prize, and a MERIT award from the U.S. National Institutes of Health. He is Fellow of the American Association for the Advancement of Science and the American Statistical Association, Associate Editor of Annals of Internal Medicine, Editor Emeritus of Epidemiology, and past Associate Editor of Biometrics, American Journal of Epidemiology, and Journal of the American Statistical Association.
The event is finished.
Local Time
- Timezone: America/New_York
- Date: Feb 28 2023
- Time: 11:00 am - 12:00 pm